What Is Data Dredging?
Data dredging alternatively referred to as data mining is a practices which involves the analyzation of large volumes of data without seeking any possible relationships between the analyzed data.
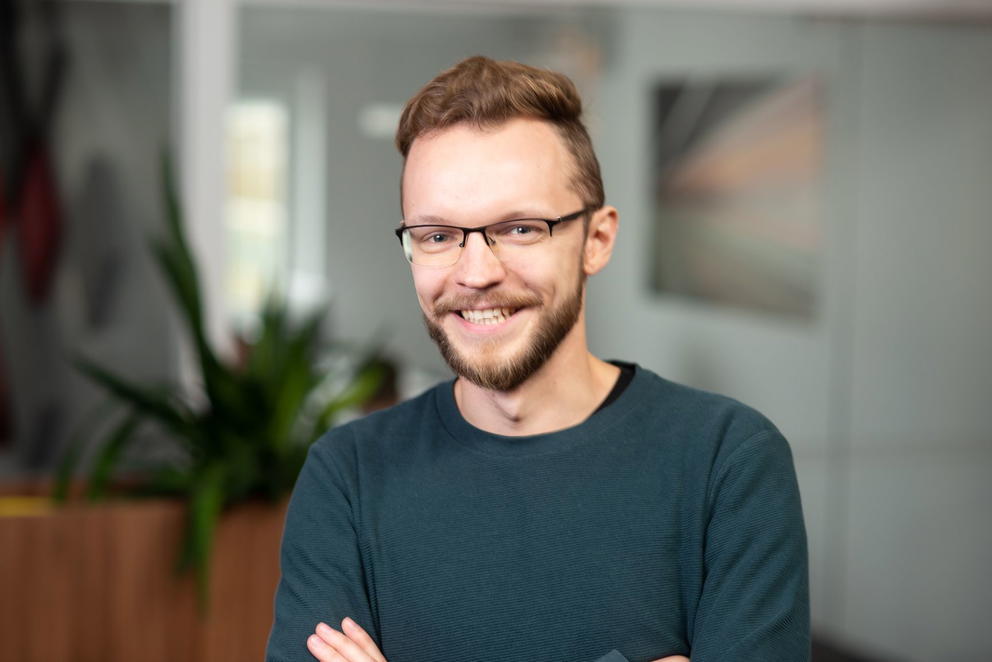
May 07 2020 ● 1 min read
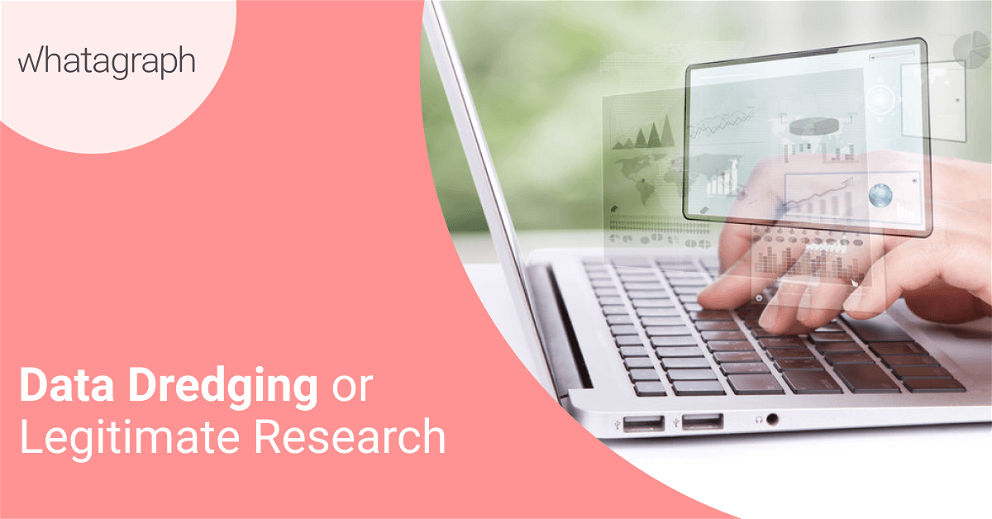
In contrast, the traditional or conventional scientific method of data dredging begins with the hypothesis and then extends through the stages of data examination.
Alternatively conducted for unethical purposes, data dredging is a data mining process that possibly circumvents the traditional techniques of data mining which may then results in premature conclusions.
In the simplest sense, data dredging is described as the act of seeking information from a set of data than it actually contains.
What Does Data Dredging Means in Your Business
Let’s imagine that you want to evaluate the fact that some subsets of your prospects or customers are more likely to upgrade than others. You then tested a specific variable or characteristics of one of your customers and the results of the test revealed that there seems to be a statistical significance.
As a modern data-savvy individual, you then begin to dredge through all the available data, while you are conserving a huge leap of information and characteristics about your customers.
Then, you proceed to develop a hypothesis from each one based on some certain criteria’s such as revenue brackets, geographic region and so forth. You continued to do this until you are able to attain a jackpot and discover that one of the hypotheses is significant. For instance, customers in North London are more likely to upgrade.
The truth is, that conclusion isn’t entirely positive. If you run that experiments continuously, chances are that you’ll discover a correlation that would seem statistically significant but it would still be false positive. Saying that particular correlation is statistically significant might be a fallacy because you are actually data dredging.
The next step is to subject sufficient number of hypothesis to tests. Averagely, you can test up to 20 hypothesis if you are utilizing the standard significance threshold of P=0.95.
Certainly, you’ll discover that some of these tested hypotheses will be statistically significant but misleading. In reality, virtually all data set with any degree of randomness has the possibility of containing some forms of false correlations.
Published on May 07 2020
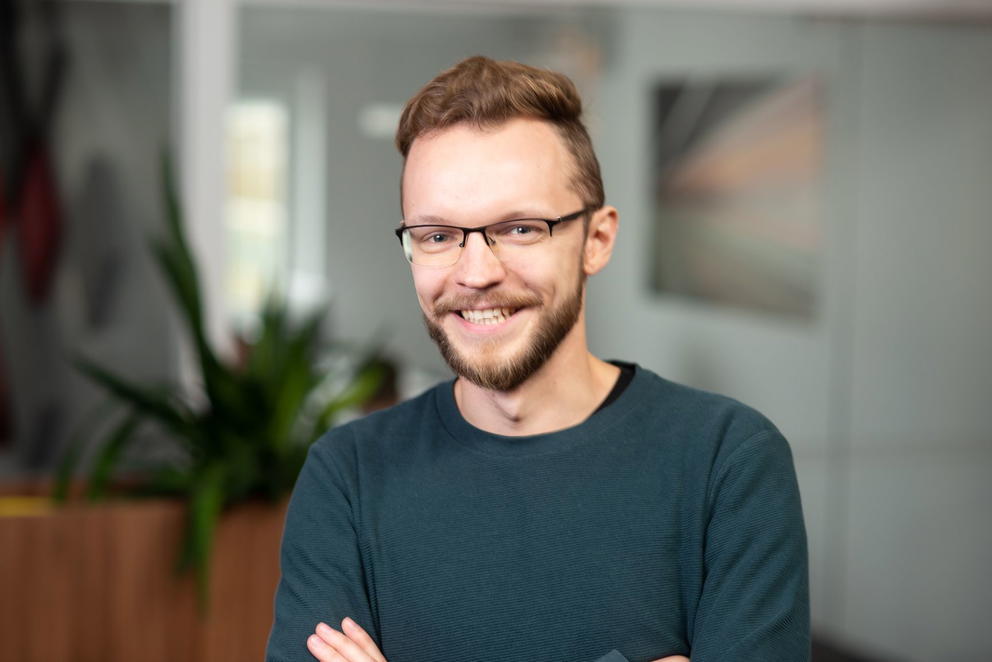
WRITTEN BY
Gintaras BaltusevičiusGintaras is an experienced marketing professional who is always eager to explore the most up-to-date issues in data marketing. Having worked as an SEO manager at several companies, he's a valuable addition to the Whatagraph writers' pool.