Data Science vs. Data Analytics
Data science and data analytics are growing at an astronomical rate and businesses use them to sift through the goldmine of data and help them make better-informed decisions.
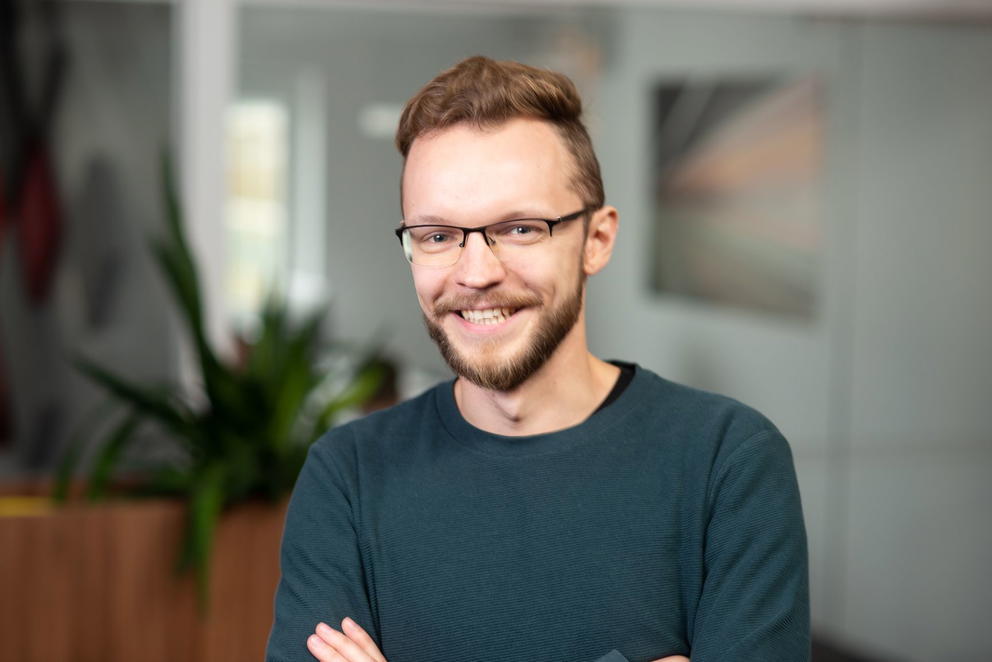
Jun 15 2020 ● 6 min read
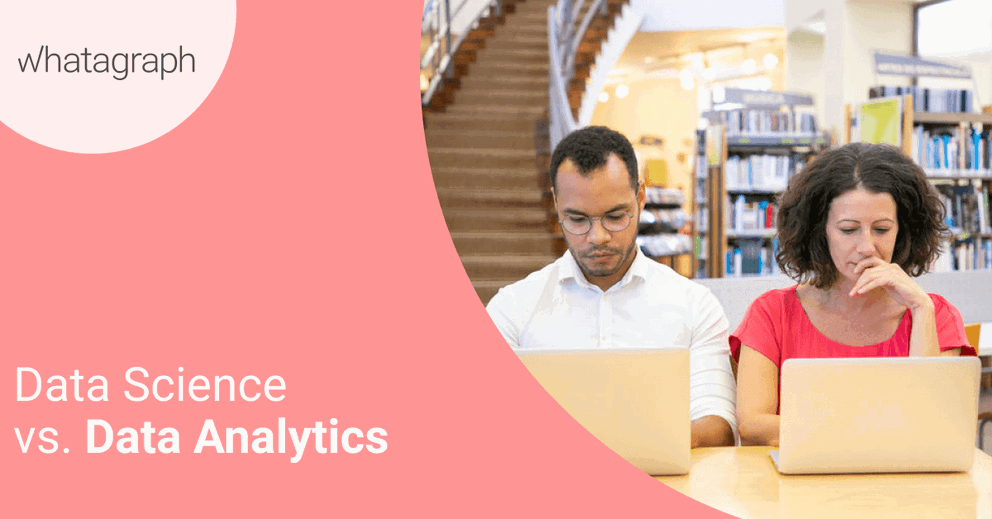
Knowing the difference between these two terms and how to use them correctly can help you run your business more efficiently and provide better solutions to the problems you face today.
Even though it may be a bit confusing at first, it’s important to understand what each of them offers and how it is unique. Before we examine their differences and find out their unique value, let’s explain both terms separately.
What Is Analytics of Data?
There’s plenty of raw data collected daily that can benefit a business if analyzed properly. This analysis of existing data to fetch, process, and organize information to get meaningful insights that can help solve current problems is called data analytics.
This term is focused on finding the answers to questions you already know that can lead to immediate improvements. It uses multiple techniques and tools to analyze big data as opposed to the manual organization of data and pure human intervention.
Here are the steps involved in it:
- Identifying the requirements and grouping of data – based on the problem or the target group and grouped in the most appropriate manner (such as location, age, lifestyle, interests, gender).
- Data collection – Collecting data from different online and offline sources like social media, physical surveys, and computers.
- Data organization – organizing it for analysis in a spreadsheet or another method.
- Removing duplicate, inconsistent, and incomplete data before analysis or data cleaning – correcting data errors and preparing data for analysis.
The data analyst is familiar with the business problem and other information and works on a familiar set of data to ensure predictive, descriptive, prescriptive, or diagnostic data analysis.
The popularity of data for analytics is continuously growing, as companies in almost every industry can benefit from it, especially in fields like finance, healthcare, tourism, retail, and hospitality industries.
What Do Data Analysts Do?
Data analysts retrieve and gather data, organize data, and use it to get meaningful solutions. They develop systems for gathering data and compile their findings into useful reports.
Every business collects data, and the responsibility of the data analyst is to figure out plenty of things from that data, such as how to reduce transportation costs, price new materials, or deal with problems that cost the business money.
They figure out how things work and try to make sense of it. These people use calculation applications and computer systems to understand their numbers. Even though numbers and facts are the starting point, it’s more important to realize what they mean and explain it with the help of charts, graphs, tables, and graphics.
A data analyst is a person who knows why the numbers are the way they are, so they advise department heads and project managers concerning specific data points and how to change or improve them.
A data analyst’s responsibilities include:
- Working with data scientists, technology teams and management to set goals;
- Collecting data;
- Data cleaning;
- Analyzing and interpreting results using certain techniques and tools;
- Recognizing patterns and trends in data sets;
- Finding new chances for process improvement;
- Making data reports for management;
- Database and data system design, creation, and maintenance;
- Fixing data-related problems and code problems.
What Is Data Science?
Data science is a large term that includes different methods and models to get information and meaningful insights from large sets of structured and raw data. It’s finding the right questions instead of answering questions you already know.
It’s connecting data points and information to identify connections that can help the business. Data science researches the world of the unknown to find new insights and patterns. It tries to make connections and build a plan for the future, unlike data analysis which checks a hypothesis.
Data science provides a new perspective into the data and shows how’s all connected, something which wasn’t seen or known previously.
The main goal of data scientists is to find the right questions and identify potential approaches of study, instead of answering specific questions. Data scientists achieve that by predicting trends, researching disconnected and disparate data sources, and discovering better wats to analyze data.
The main components of data science include:
- Statistics – using mathematical methods to collect, analyze, interpret, and present data;
- Visualization of data – presenting the results in the form of easy-to-understand charts, diagrams, and graphs that highlight the key takeaways and help for a better and quicker decision making;
- Machine learning – using intelligent algorithms that are capable of learning on their own and predicting human behavior.
What Do Data Scientists Do?
Data scientists try to understand business stakeholders’ goals and find out how to use data to reach those goals. Their power lies in their deep understanding of algorithms and statistics, hacking and programming, and communication skills.
Data scientists design data modeling processes, create predictive models and algorithms to extract the necessary data for a particular business, assist with data analysis, and share insights with peers.
They locate and define potential problems in business from different unrelated sources and obtain data from them. After the data analytics, a model is created and tested for accuracy.
Some of their responsibilities include:
- Finding the right questions to ask to start the discovery process;
- Collecting data;
- Data processing and cleaning;
- Integrating and storing data;
- Investigating data;
- Choosing the right algorithms and models;
- Applying data science techniques and methods such as statistical modeling, machine learning, and AI;
- Measuring and improving results;
- Showing final results and making the necessary changes based on feedback.
Does Data analysis and data science are the same?
The biggest difference is the scope. The first term encompasses data analytics, machine learning, data mining, and other related disciplines that are used to mine large data sets.
Data analytics, on the other hand, is much more focused than data science and is a part of the larger process. It’s focused on finding meaningful insights that can be applied right away based on existing questions.
When it comes to the exploration process, data science doesn’t deal with answering already known questions but parses through humongous datasets in rather unstructured ways to uncover insights.
Data analytics, however, has specific questions in mind that help it to focus on finding the right answers based on existing data.
Overall, data science generates broader insights that focus on finding new questions that you might not have been aware they need to be answered to drive innovation, while data analytics focuses on finding answers to already known questions for better decision making.
Data science vs. data analytics main differences:
- The multidisciplinary field of data science includes data analytics, machine learning, domain expertise, computer science, and mathematics. Data analytics is part of data science where information is processed, organized, and analyzed to find solutions to problems;
- The scope of data science is macro, while that od data analytics is micro;
- The input in data science is unstructured or raw data that needs to be organized and cleaned to be analyzed. On the other hand, the input in data analytics is structured data on which data visualization and design principles are applied;
- The purpose of data science is to discover and define new business problems to drive innovation, while that of data analytics is to find the best solution or answer to an already known problem;
- Data science discovers new and unknown problems and finds solutions to them by converting data into use cases and business stories. In data analytics, however, the data is only analyzed and interpreted without creating a roadmap;
- Data science requires knowledge of machine learning, advanced statistics, data modeling, and basic knowledge of SAS, Python/R, SQL, and other programming languages. Data analytics, on the other hand, requires knowledge of BI tools, databases like SQL, and statistics;
- Data science involves machine learning, artificial intelligence, and search engine exploration, while data analytics involves only analytical techniques using statistical techniques and tools;
- Data science is used for internet research, recommender systems, digital marketing, speech recognition, and image recognition, while data analytics is used in domain areas such as gaming, travel and tourism, finance, healthcare, etc;
- Data science is a more paid job than data analytics.
To Sum Up
Even though we’ve established that data science and data analytics are different, their functions are extremely interconnected. So, it’s better to view them as parts of a whole that are important for a business to move in the right direction and make better decisions by understanding, analyzing, and reviewing the information you have.
Published on Jun 15 2020
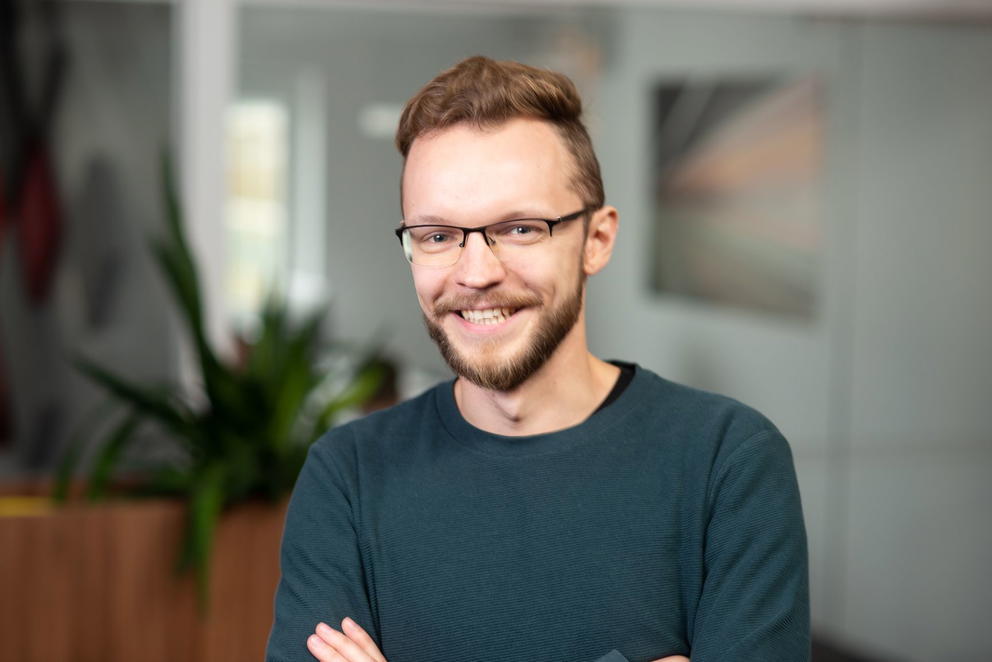
WRITTEN BY
Gintaras BaltusevičiusGintaras is an experienced marketing professional who is always eager to explore the most up-to-date issues in data marketing. Having worked as an SEO manager at several companies, he's a valuable addition to the Whatagraph writers' pool.